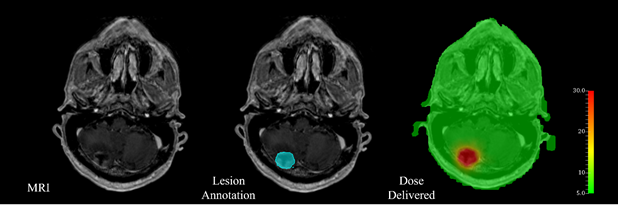
Notice: Function Pods Templates was called incorrectly. Pod Template PHP code is no longer actively supported and will be completely removed in Pods 3.3 Please see Debugging in WordPress for more information. (This message was added in version 3.0.) in /var/www/html/wp-includes/functions.php on line 6085
Deprecated: Pod Template PHP code has been deprecated, please use WP Templates instead of embedding PHP. has been deprecated since Pods version 2.3 with no alternative available. in /var/www/html/wp-content/plugins/pods/includes/general.php on line 1037
Brain-TR-GammaKnife | Brain Tumor Recurrence Prediction after Gamma Knife Radiotherapy from MRI and Related DICOM-RT: An Open Annotated Dataset and Baseline Algorithm
DOI: 10.7937/xb6d-py67 | Data Citation Required | Image Collection
Location | Species | Subjects | Data Types | Cancer Types | Size | Status | Updated | |
---|---|---|---|---|---|---|---|---|
Brain | Human | 47 | RTSTRUCT, MR, RTDOSE, Segmentation, Other | Brain Cancer | Clinical, Image Analyses, Software/Source Code | Limited, Complete | 2023/03/21 |
Summary
Here we release a brain cancer MRI dataset with the companion Gamma Knife treatment planning and follow-up data for the purpose of tumor recurrence prediction. The dataset consists of 47 subjects. A total of 244 lesions were collected with annotations. The dataset contains original patient MRI images (in DICOM format), radiation therapy structure data (in DICOM and NRRD format), code, and clinical information. First, dose MRI images were resampled to original MRI spacing via a linear transformation. Second, each region in each patient's MRI was extracted and cropped out; note that one patient may have multiple lesions and/or multiple imaging sessions. Third, the corresponding radiation dose information was cropped out to the resampled aligned lesion mask. In this way, each lesion MRI is paired with its radiation dose MRI. The release of this dataset is expected to contribute to the development of automated brain tumor recurrence prediction algorithms. Prediction and identification of tumor recurrence are critical for brain cancer treatment design and planning. Stereotactic radiation therapy delivered with Gamma Knife has been developed as one of the common treatment approaches in addition to others by delivering radiation that accurately to the tumor while minimizing toxicity to nearby healthy tissues. Artificial intelligence (AI)-based research has shown great potential in brain tumor MRI analysis recently with its effective data-driven feature extraction and recognition capabilities. Here we release a brain cancer MRI dataset with the companion Gamma Knife treatment planning and follow-up data for the purpose of tumor recurrence prediction. The dataset contains original patient MRI images, radiation therapy data, and additional clinical information. The included MRI series are T1 MPRAGE with Gadolinium contrast acquired on a 1.5 T Siemens Magnetom scanner for Gamma Knife treatment for brain metastasis. This public dataset retrospectively involved a total of 47 brain cancer patients, organized based on patient-level, course-level, and lesion-level, respectively. Patient-level keeps all the data related to a specific patient altogether. Each course-level treatment data has been stored for each patient. Among all the patients, 17 patients received more than 1 course of treatment, 7 received more than 2 courses, and 1 patient received up to 8 courses in total. Regarding to the lesion-level, a total of 244 lesions are collected with annotations. 221 lesions are stable, and 23 are recurrence, which makes the dataset imbalance from the lesion-level perspective. Lesion annotations are provided, and inclusive preprocessing steps have been specified to simplify the usage of this dataset. The release of this dataset is expected to contribute to future development of automated brain tumor recurrence prediction algorithms and promote the clinical implementations associated with AI-based computer-aided diagnosis field. This dataset was collected retrospectively under IRB-approval (2017-0266) from a clinical database of patients treated for brain metastases with Gamma Knife radiation therapy at the University of Mississippi Medical Center (UMMC). The dataset consisted of 47 brain cancer with 21 males and 26 females who developed brain metastases for various primary tumors and then underwent Gamma Knife stereotactic radiation therapy in an effort to control the identified brain lesions. A total of 244 lesions are collected with annotations. Additionally, these patients participated in treatment follow-up during which the response to treatment could be adequately assessed to identify recurring lesions and those that responded to treatment by stopping growth or even shrinking. A keyed spreadsheet stored various other relevant clinical information as well as how many treatment sessions were used to deliver the dose for each target. It should be noted that each patient may have undergone more than one treatment course as they developed new lesions and/or treated lesions recurred. For each patient, the treatment targets were identified and delineated through collaboration between radiation oncologists and neurosurgeons with validation from neuroradiologists. In preparation for the dataset, an MRI was acquired on a 1.5 T Siemens Magnetom scanner. The included MRI series are T1 MPRAGE with Gadolinium contrast and a voxel size of 1 × 1 × 1 mm which acts as the primary planning dataset for Gamma Knife treatment for brain metastases. For each patient, the treatment planning DICOM data was collected in the MRI dataset with its accompanying RTStruct, which indicates the lesions that were targeted using a given MRI. The RTDose information provides how much and where dose was deposited for each respective lesion/target. The treatment sessions were recorded to describe the dose delivery for a target represented in the RTDose as it may have been delivered in as few as 1, but maybe as many as 5 treatment sessions. Additionally, each patient may have undergone more than one treatment course as they developed new lesions and/or treated lesions recurred. The dose units are in Gray (Gy, AKA J/kg) which is typical in radiation therapy. The dose was calculated using the TMR10 algorithm in the GammaPlan® (version 11) software which does not consider tissue heterogeneity in the calculation but is consistent with previous Gamma Knife treatment practices across many institutions. Treatment was ultimately delivered on the Gamma Knife Icon® at the same institution. After collection, all DICOM data (MRI images, RTStruct, and RTDose) was fully anonymized removing all protected health information and treatment-related dates. The anonymization process included mapping them to identifiers that match the clinical information in the database spreadsheet. In this dataset, both original and preprocessed data are available for both clinical and engineering researchers. For preprocessed images, we specify a series of preprocessing steps that have been conducted. First, dose MRI images were resampled to the same spacing as the original patient MRI via a linear transformation process because the raw MRI and dose files share not only different dimensions but incompatible actual spacing. Second, each lesion region in each patient's MRI was extracted and cropped out based on the patient's lesion mask. It is noted that one patient may have multiple lesions. Third, the corresponding radiation dose information was also cropped out using the same lesion mask because the masks are shared through the same coordinate after resampling. In this way, each lesion MRI is paired with its radiation dose MRI. For any AI model training or test, the voxel values are recommended to be normalized to the range of 0 to 1 in each cropped lesion and dose MRI pair. Resizing is another necessary preprocessing step to keep the cropped training and test samples in the same dimension. In our dataset, we kept the original dimension of the cropped lesions. Since the dataset is imbalanced in terms of lesion-level, data augmentation techniques are suggested to be employed before model training. The aim of this dataset is to facilitate the development and evolution of automated tumor recurrence identification. Further potential applications based on this dataset include radiation treatment planning, evaluating and refining, and automated dose delivery planning. AI research community may take advantage of this public data resource to achieve promising results and promote the implementation of artificial intelligence-assist tumor recurrence prediction. Different preprocessing procedures in terms of various focuses and implementation can be used, and more advanced AI frameworks and techniques are expected to be developed.Introduction
Data Records and Population
Image Acquisition
Data Preprocessing and Usage
Data Access
Some data in this collection contains images that could potentially be used to reconstruct a human face. To safeguard the privacy of participants, users must sign and submit a TCIA Restricted License Agreement to help@cancerimagingarchive.net before accessing the data.
Version 1: Updated 2023/03/21
Title | Data Type | Format | Access Points | Subjects | License | |||
---|---|---|---|---|---|---|---|---|
Images, Segmentations, Radiation Therapy Structures/Doses | RTSTRUCT, MR, RTDOSE | DICOM | Download requires NBIA Data Retriever |
47 | 76 | 228 | 16,792 | TCIA Restricted |
Images, Segmentations, Radiation Therapy Structures/Doses | MR, Segmentation, RTDOSE | NRRD | Download requires IBM-Aspera-Connect plugin |
47 | 399 | TCIA Limited (contact Support) | ||
Clinical data | XLSX | CC BY 4.0 | ||||||
Clinical Data Name Glossary | Other | DOCX | CC BY 4.0 | |||||
Clinical Data Column Description | Other | DOCX | CC BY 4.0 |
Additional Resources for this Dataset
The following external resources have been made available by the data submitters. These are not hosted or supported by TCIA, but may be useful to researchers utilizing this collection.
- Source code: https://github.com/siolmsstate/brain_mri
Citations & Data Usage Policy
Data Citation Required: Users must abide by the TCIA Data Usage Policy and Restrictions. Attribution must include the following citation, including the Digital Object Identifier:
Data Citation |
|
Wang, Y., Duggar, W.N., Caballero, D.M., Vengaloor Thomas, T., Adari, N., Mundra, E.K., Wang, H. (2023) Brain Tumor Recurrence Prediction after Gamma Knife Radiotherapy from MRI and Related DICOM-RT: An Open Annotated Dataset and Baseline Algorithm (Brain-TR-GammaKnife) [Dataset]. The Cancer Imaging Archive. DOI: https://doi.org/10.7937/xb6d-py67 |
Detailed Description
Note:
Individual RTDose series may have a different study date (DICOM tag (0008,0020) than series date (DICOM tag (0008,0021)) . If this is seen, the PI has edited those files so that the SERIES_DATE correctly indicated the treatment start date. Therefore, because the study and series date offsets are consistent, then the difference is meaningful in how long of a gap existed between imaging and treatment.
Acknowledgements
We would like to acknowledge the individuals and institutions that have provided data for this collection:
- The raw MRI and DICOM-RT data were collected and supported by the Department of Radiation Oncology at The University of Mississippi Medical Center (UMMC).
- The MRI and DICOM-RT data matching, preprocessing, and AI-based validation were supported by the Department of Industrial and Systems Engineering at Mississippi State University (MSState).
Related Publications
Publications by the Dataset Authors
The authors recommended this paper as the best source of additional information about this dataset:
Wang, Y., Duggar, W.N., Caballero, D.M., Vengaloor Thomas, T., Adari, N., Mundra, E.K., Wang, H. (2023) A brain MRI dataset and baseline evaluations for tumor recurrence prediction after Gamma Knife radiotherapy. Sci Data 10, 785 (2023). DOI: https://doi.org/10.1038/s41597-023-02683-1
The Collection authors suggest the below will give context to this dataset:
- This dataset has been used in the 2022 Student Data Analytics Competition by Institute of Industrial and Systems Engineers. https://www.iise.org/Details.aspx?id=50000
Research Community Publications
TCIA maintains a list of publications which leverage TCIA data. If you have a manuscript you’d like to add please contact TCIA’s Helpdesk.