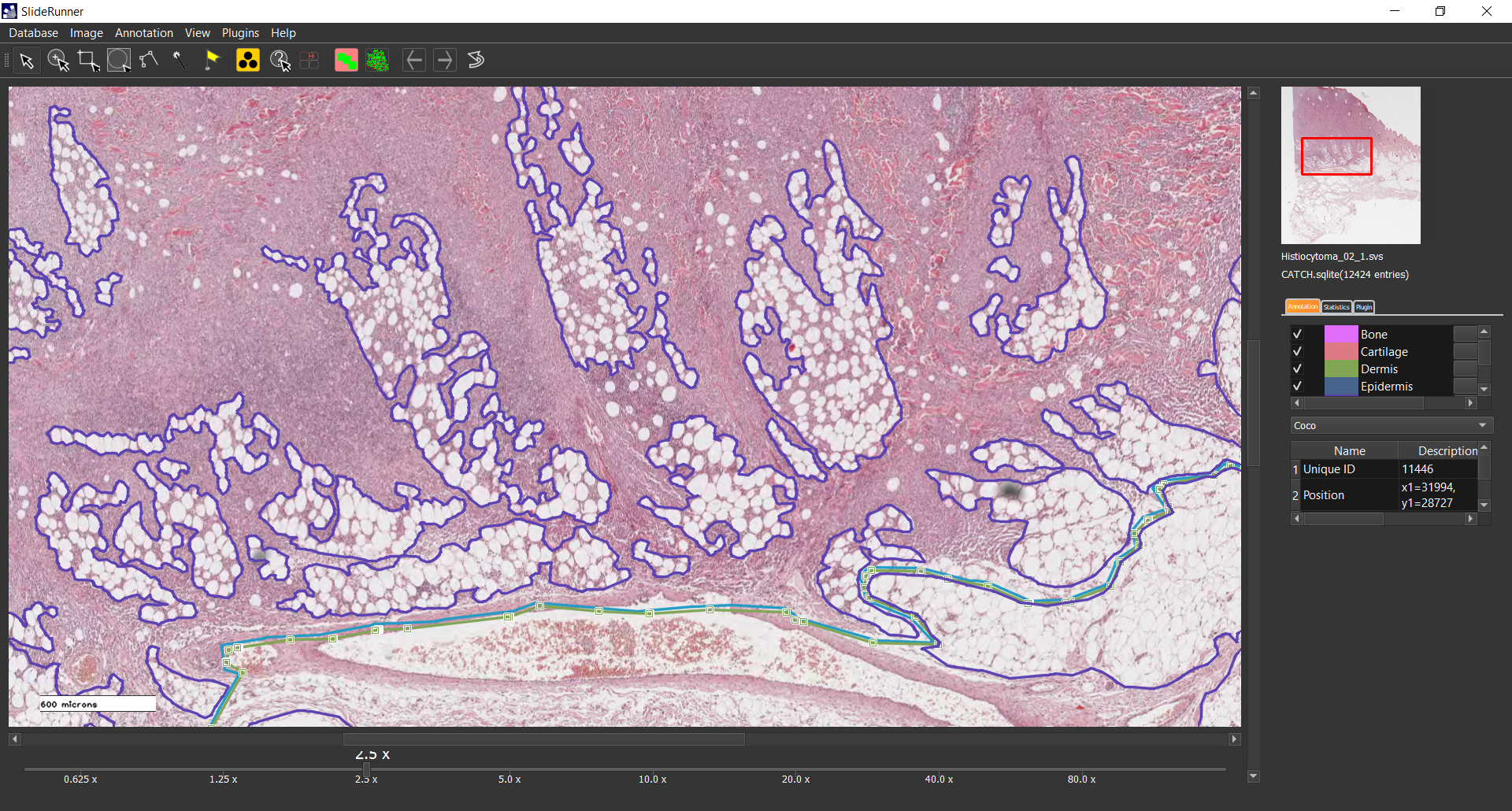
Notice: Function Pods Templates was called incorrectly. Pod Template PHP code is no longer actively supported and will be completely removed in Pods 3.3 Please see Debugging in WordPress for more information. (This message was added in version 3.0.) in /var/www/html/wp-includes/functions.php on line 6085
Deprecated: Pod Template PHP code has been deprecated, please use WP Templates instead of embedding PHP. has been deprecated since Pods version 2.3 with no alternative available. in /var/www/html/wp-content/plugins/pods/includes/general.php on line 1037
CATCH | CAnine CuTaneous Cancer Histology Dataset
DOI: 10.7937/TCIA.2M93-FX66 | Data Citation Required | Image Collection
Location | Species | Subjects | Data Types | Cancer Types | Size | Status | Updated | |
---|---|---|---|---|---|---|---|---|
Skin | Canine | 282 | Histopathology | Skin Cancer | Image Analyses, Software/Source Code | Public, Complete | 2022/01/12 |
Summary
We present a large-scale dataset of 350 histologic samples of seven different canine cutaneous tumors. All samples were obtained through surgical resection due to neoplastic indicators and were selected retrospectively from the biopsy archive of the Institute for Veterinary Pathology of the Freie Universität Berlin according to sufficient tissue preservation and presence of characteristic histologic features for the corresponding tumor subtypes. Samples were stained with a routine Hematoxylin & Eosin dye and digitized with two Leica linear scanning systems at a resolution of 0.25 um/pixel. Together with the 350 whole slide images, we provide a database consisting of 12,424 polygon annotations for six non-neoplastic tissue classes (epidermis, dermis, subcutis, bone, cartilage, and a joint class of inflammation and necrosis) and seven tumor classes (melanoma, mast cell tumor, squamous cell carcinoma, peripheral nerve sheath tumor, plasmacytoma, trichoblastoma, and histiocytoma). The polygon annotations were generated using the open source software SlideRunner (https://github.com/DeepPathology/SlideRunner). Within SlideRunner, users can view whole slide images (WSIs) and zoom through their magnification levels. Using multiple clicks or click-and-drag, the pathologist annotated polygons for 13 classes (epidermis, dermis, subcutis, bone, cartilage, a joint class of inflammation and necrosis, melanoma, mast cell tumor, squamous cell carcinoma, peripheral nerve sheath tumor, plasmacytoma, trichoblastoma, and histiocytoma) on 287 WSIs. The remaining WSIs were annotated by three medical students in their 8th semester supervised by the leading pathologist who later reviewed these annotations for correctness and completeness. Due to the large size of the dataset and the extensive annotations, it provides a good basis for segmentation and classification algorithms based on supervised learning. Previous work [1-4] has shown, that due to various homologies between the species, canine cutaneous tissue can serve as a model for human samples. Prouteau et al. have published an extensive comparison of the two species especially for cutaneous tumors and include homologies between canine and human oncology regarding "clinical and histological appearance, biological behavior, tumor genetics, molecular pathways and targets, and response to therapies" [1]. Ranieri et al. highlight that pet dogs and humans share many environmental risk factors and show the highest risk for cancer development at similar points of time respective to their life spans [2]. Both, Ranieri et al. and Pinho et al. highlight the potential of using insights from experiments on canine samples for developing human cancer treatments [2,3]. From a technical perspective, Aubreville et al. have shown that canine samples can be used to aid human cancer research through the use of transfer learning methods [4]. Potential users of the dataset can load the SQLite database into their custom installation of SlideRunner and adapt or extend the database with custom annotations. Furthermore, we converted the annotations to the COCO JSON format, which is commonly used by computer scientists for training neural networks. Its pixel-level annotations can be used for supervised segmentation algorithms as opposed to datasets that only provide clinical data on slide level.References
Data Access
Version 1: Updated 2022/01/12
Title | Data Type | Format | Access Points | Subjects | License | |||
---|---|---|---|---|---|---|---|---|
Tissue Slide Images | Histopathology | SVS | Download requires IBM-Aspera-Connect plugin |
282 | 350 | CC BY 4.0 | ||
Annotations | JSON | CC BY 4.0 | ||||||
Annotations | ZIP and SQLITE | CC BY 4.0 |
Additional Resources for this Dataset
The following external resources have been made available by the data submitters. These are not hosted or supported by TCIA, but may be useful to researchers utilizing this collection.
- The polygon annotations were generated using the open source software SlideRunner (https://github.com/DeepPathology/SlideRunner).
Citations & Data Usage Policy
Data Citation Required: Users must abide by the TCIA Data Usage Policy and Restrictions. Attribution must include the following citation, including the Digital Object Identifier:
Data Citation |
|
Wilm, F., Fragoso, M., Marzahl, C., Bertram, C., Klopfleisch, R., Maier, A., Aubreville, M., & Breininger, K. (2022). CAnine CuTaneous Cancer Histology Dataset (Version 1) [Data set]. The Cancer Imaging Archive. https://doi.org/10.7937/TCIA.2M93-FX66 |
Acknowledgements
The authors would like to thank everyone who contributed to the creation of this dataset through providing samples, annotations or technical support.
Related Publications
Publications by the Dataset Authors
The authors recommended this paper as the best source of additional information about this dataset:
The Collection authors suggest the below will give context to this dataset:
- Prouteau, & André. (2019). Canine Melanomas as Models for Human Melanomas: Clinical, Histological, and Genetic Comparison. In Genes (Vol. 10, Issue 7, p. 501). MDPI AG. https://doi.org/10.3390/genes10070501
- Ranieri, G., Gadaleta, C. D., Patruno, R., Zizzo, N., Daidone, M. G., Hansson, M. G., Paradiso, A., & Ribatti, D. (2013). A model of study for human cancer: Spontaneous occurring tumors in dogs. Biological features and translation for new anticancer therapies. In Critical Reviews in Oncology/Hematology (Vol. 88, Issue 1, pp. 187–197). Elsevier BV. https://doi.org/10.1016/j.critrevonc.2013.03.005
- Pinho, S. S., Carvalho, S., Cabral, J., Reis, C. A., & Gärtner, F. (2012). Canine tumors: a spontaneous animal model of human carcinogenesis. In Translational Research (Vol. 159, Issue 3, pp. 165–172). Elsevier BV. https://doi.org/10.1016/j.trsl.2011.11.005
- Aubreville, M., Bertram, C. A., Donovan, T. A., Marzahl, C., Maier, A., & Klopfleisch, R. (2020). A completely annotated whole slide image dataset of canine breast cancer to aid human breast cancer research. In Scientific Data (Vol. 7, Issue 1). Springer Science and Business Media LLC. https://doi.org/10.1038/s41597-020-00756-z
Research Community Publications
TCIA maintains a list of publications which leverage TCIA data. If you have a manuscript you’d like to add please contact TCIA’s Helpdesk.
- Oza, P. R., Sharma, P., & Patel, S. (2022). A Transfer Representation Learning Approach for Breast Cancer Diagnosis from Mammograms using EfficientNet Models. In Scalable Computing: Practice and Experience (Vol. 23, Issue 2, pp. 51–58). Scalable Computing: Practice and Experience. https://doi.org/10.12694/scpe.v23i2.1975