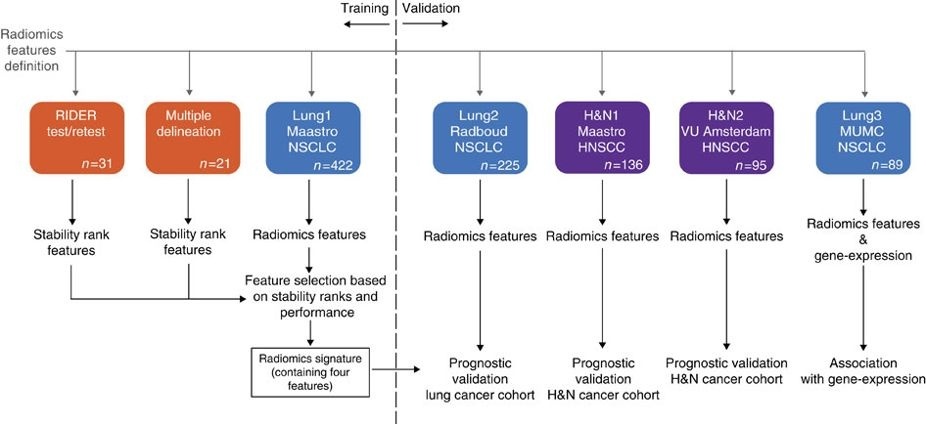
Notice: Function Pods Templates was called incorrectly. Pod Template PHP code is no longer actively supported and will be completely removed in Pods 3.3 Please see Debugging in WordPress for more information. (This message was added in version 3.0.) in /var/www/html/wp-includes/functions.php on line 6085
Deprecated: Pod Template PHP code has been deprecated, please use WP Templates instead of embedding PHP. has been deprecated since Pods version 2.3 with no alternative available. in /var/www/html/wp-content/plugins/pods/includes/general.php on line 1037
HEAD-NECK-RADIOMICS-HN1 | HEAD-NECK-RADIOMICS-HN1
DOI: 10.7937/tcia.2019.8kap372n | Data Citation Required | Image Collection
Location | Species | Subjects | Data Types | Cancer Types | Size | Status | Updated | |
---|---|---|---|---|---|---|---|---|
Head-Neck | Human | 137 | SEG, RTSTRUCT, CT, PT, Demographic, Follow-Up, Other | Head and Neck Cancer | Clinical | Limited, Complete | 2020/07/29 |
Summary
This collection contains clinical data and computed tomography (CT) from 137 head and neck squamous cell carcinoma (HNSCC) patients treated by radiotherapy. For these patients a pre-treatment CT scan was manual delineated by an experienced radiation oncologist of the 3D volume of the gross tumor volume. This dataset refers to the "H&N1" dataset of the study published in Nature Communications (http://doi.org/10.1038/ncomms5006). At time of previous publication, images of one subject had been unintentionally overlooked. In short, the publication used a radiomics approach to computed tomography data of 1,019 patients with lung or head-and-neck cancer. Radiomics refers to the comprehensive quantification of tumor phenotypes by applying a large number of quantitative image features. In the published analysis, 440 features quantifying tumor image intensity, shape, and texture were extracted. We found that a large number of radiomic features have prognostic power in independent data sets, many of which were not identified as significant before. Radiogenomics analysis revealed that a prognostic radiomic signature, capturing intra-tumor heterogeneity, was associated with underlying gene-expression patterns. These data suggest that radiomics identifies a general prognostic phenotype existing in both lung and head-and-neck cancer. This may have a clinical impact as imaging is routinely used in clinical practice, providing an unprecedented opportunity to improve decision-support in cancer treatment at low cost. This dataset is provided as open access to support repeatability and reproducibility of research in radiomics. This dataset will be the subject of an upcoming article addressing FAIR radiomics practices to support transparency, harmonization and collaboration on radiomics. From version 2 (release date 09/20/2019) onwards we included the primary neoplasm gross tumour volume delineations in DICOM SEGMENTATION as well as DICOM RTSTRUCT files that accompanied the DICOM axial images. This dataset is provided as open access to support repeatability and reproducibility of research in radiomics. This dataset will be the subject of an upcoming article addressing FAIR radiomics practices to support transparency, harmonization and collaboration on radiomics. Other data sets in the Cancer Imaging Archive that were used in the same study published in Nature Communications: NSCLC-Radiomics, NSCLC-Radiomics-Genomics, NSCLC-Radiomics-Interobserver1, RIDER-LungCT-Seg.
Data Access
Some data in this collection contains images that could potentially be used to reconstruct a human face. To safeguard the privacy of participants, users must sign and submit a TCIA No Commercial Limited Access License to help@cancerimagingarchive.net before accessing the data.
Version 3: Updated 2020/07/29
Added the chemotherapy schedule to the clinical data; one extra column added which is “chemotherapy_given”.
Added data dictionary for clinical data.
Title | Data Type | Format | Access Points | Subjects | License | |||
---|---|---|---|---|---|---|---|---|
Images, Segmentations, and Radiation Therapy Structures | SEG, RTSTRUCT, CT, PT | DICOM | Download requires NBIA Data Retriever |
137 | 137 | 486 | 28,918 | TCIA No Commercial Limited |
Clinical Data | Demographic, Follow-Up | ZIP and CSV | 137 | CC BY-NC 3.0 | ||||
Data Dictionary | Other | TXT | CC BY-NC 3.0 |
Citations & Data Usage Policy
Data Citation Required: Users must abide by the TCIA Data Usage Policy and Restrictions. Attribution must include the following citation, including the Digital Object Identifier:
Data Citation |
|
Wee, L., & Dekker, A. (2019). Data from HEAD-NECK-RADIOMICS-HN1 [Data set]. The Cancer Imaging Archive. https://doi.org/10.7937/tcia.2019.8kap372n |
Acknowledgements
We would like to acknowledge the individuals and institutions that have provided data for this collection:
- Leonard Wee, MAASTRO (Dept of Radiotherapy), Maastricht University Medical Centre+, Maastricht, Limburg, The Netherlands.
- Frank Hoebers, MAASTRO (Dept of Radiotherapy), Maastricht University Medical Centre+, Maastricht, Limburg, The Netherlands.
- Andre Dekker, MAASTRO (Dept of Radiotherapy), Maastricht University Medical Centre+, Maastricht, Limburg, The Netherlands.
- Hugo Aerts, Computational Imaging and Bioinformatic Laboratory, Dana-Farber Cancer Institute & Harvard Medical School, Boston, Massachusetts, USA.
Related Publications
Publications by the Dataset Authors
The authors recommended this paper as the best source of additional information about this dataset:
Aerts HJWL, Velazquez ER, Leijenaar RTH, Parmar C, Grossmann P, Carvalho S, Bussink J, Monshouwer R, Haibe-Kains B, Rietveld D, Hoebers F, Rietbergen MM, Leemans CR, Dekker A, Quackenbush J, Gillies RJ, Lambin P. Decoding Tumour Phenotype by Noninvasive Imaging Using a Quantitative Radiomics Approach, Nature Communications, Volume 5, Article Number 4006, June 03, 2014. DOI: http://doi.org/10.1038/ncomms5006
No publications by dataset authors were found.
Research Community Publications
TCIA maintains a list of publications which leverage our data. If you have a manuscript you’d like to add please contact TCIA’s Helpdesk.
- Bielak, L., Wiedenmann, N., Berlin, A., Nicolay, N. H., Gunashekar, D. D., Hagele, L., . . . Bock, M. (2020). Convolutional neural networks for head and neck tumor segmentation on 7-channel multiparametric MRI: a leave-one-out analysis. Radiat Oncol, 15(1), 181. doi:https://doi.org/10.1186/s13014-020-01618-z
- Choi, Y., Nam, Y., Jang, J., Shin, N. Y., Ahn, K. J., Kim, B. S., . . . Kim, M. S. (2020). Prediction of Human Papillomavirus Status and Overall Survival in Patients with Untreated Oropharyngeal Squamous Cell Carcinoma: Development and Validation of CT-Based Radiomics. AJNR Am J Neuroradiol, 41(10), 1897-1904. doi: https://doi.org/10.3174/ajnr.A6756
- Gifford, R. C. (2022). Deep Learning Architecture to Improve Edge Accuracy of Auto-Contouring for Head and Neck Radiotherapy. (Master of Science MS). Ohio State UNiversity, Retrieved from http://rave.ohiolink.edu/etdc/view?acc_num=osu1658317931555616
- Giraud, P., Giraud, P., Nicolas, E., Boisselier, P., Alfonsi, M., Rives, M., . . . Chajon, E. (2021). Interpretable Machine Learning Model for Locoregional Relapse Prediction in Oropharyngeal Cancers. Cancers, 13(1), 57. doi:https://doi.org/10.3390/cancers13010057
- Kalendralis, P. (2022). Artificial intelligence applications in radiotherapy: The role of the FAIR data principles. (Ph.D. Dissertation). Maastricht University ,The Netherlands, Available from https://doi.org/10.26481/dis.20221010pk
- Kalendralis, P., Shi, Z., Traverso, A., Choudhury, A., Sloep, M., Zhovannik, I., . . . Wee, L. (2020). FAIR-compliant clinical, radiomics and DICOM metadata of RIDER, interobserver, Lung1 and head-Neck1 TCIA collections. Med Phys. doi:https://doi.org/10.1002/mp.14322
- La Greca Saint-Esteven, A., Bogowicz, M., Konukoglu, E., Riesterer, O., Balermpas, P., Guckenberger, M., . . . van Timmeren, J. E. (2022). A 2.5D convolutional neural network for HPV prediction in advanced oropharyngeal cancer. Comput Biol Med, 142, 105215. doi:https://doi.org/10.1016/j.compbiomed.2022.105215
- Li, J., Qiu, Z., Zhang, C., Chen, S., Wang, M., Meng, Q., . . . Zhang, X. (2022). ITHscore: comprehensive quantification of intra-tumor heterogeneity in NSCLC by multi-scale radiomic features. Eur Radiol. doi:https://doi.org/10.1007/s00330-022-09055-0
- Lombardo, E., Kurz, C., Marschner, S., Avanzo, M., Gagliardi, V., Fanetti, G., . . . Landry, G. (2021). Distant metastasis time to event analysis with CNNs in independent head and neck cancer cohorts. Sci Rep, 11(1), 6418. doi:https://doi.org/10.1038/s41598-021-85671-y
- Lv, W., Zhou, Z., Peng, J., Peng, L., Lin, G., Wu, H., . . . Lu, L. (2023). Functional-structural Sub-region Graph Convolutional Network (FSGCN): Application to the Prognosis of Head and Neck Cancer with PET/CT imaging. Computer Methods and Programs in Biomedicine. doi:https://doi.org/10.1016/j.cmpb.2023.107341
- Moitra, D., & Mandal, R. K. (2022). Classification of malignant tumors by a non-sequential recurrent ensemble of deep neural network model. Multimed Tools Appl, 1-19. doi:https://doi.org/10.1007/s11042-022-12229-z
- Zhinan, L., Wei, Z., Yudi, Y., Yabing, D., Yuanzhe, X., & Xiulan, L. (2022). Prediction of Human Papillomavirus (HPV) Status in Oropharyngeal Squamous Cell Carcinoma Based on Radiomics and Machine Learning Algorithms: A Multi-Cohort Study. Systematic Reviews in Pharmacy.
Previous Versions
Version 2: Updated 2019/09/20
Added DICOM Segmentations
Title | Data Type | Format | Access Points | License | ||||
---|---|---|---|---|---|---|---|---|
Images | DICOM | Download requires NBIA Data Retriever |
||||||
Clinical Data | CSV |
Version 1: Updated 2019/07/25
Title | Data Type | Format | Access Points | License | ||||
---|---|---|---|---|---|---|---|---|
Images | DICOM | Download requires NBIA Data Retriever |
||||||
Clinical Data | CSV |